The Potential of Generative AI in Financial Services: A Comprehensive Analysis
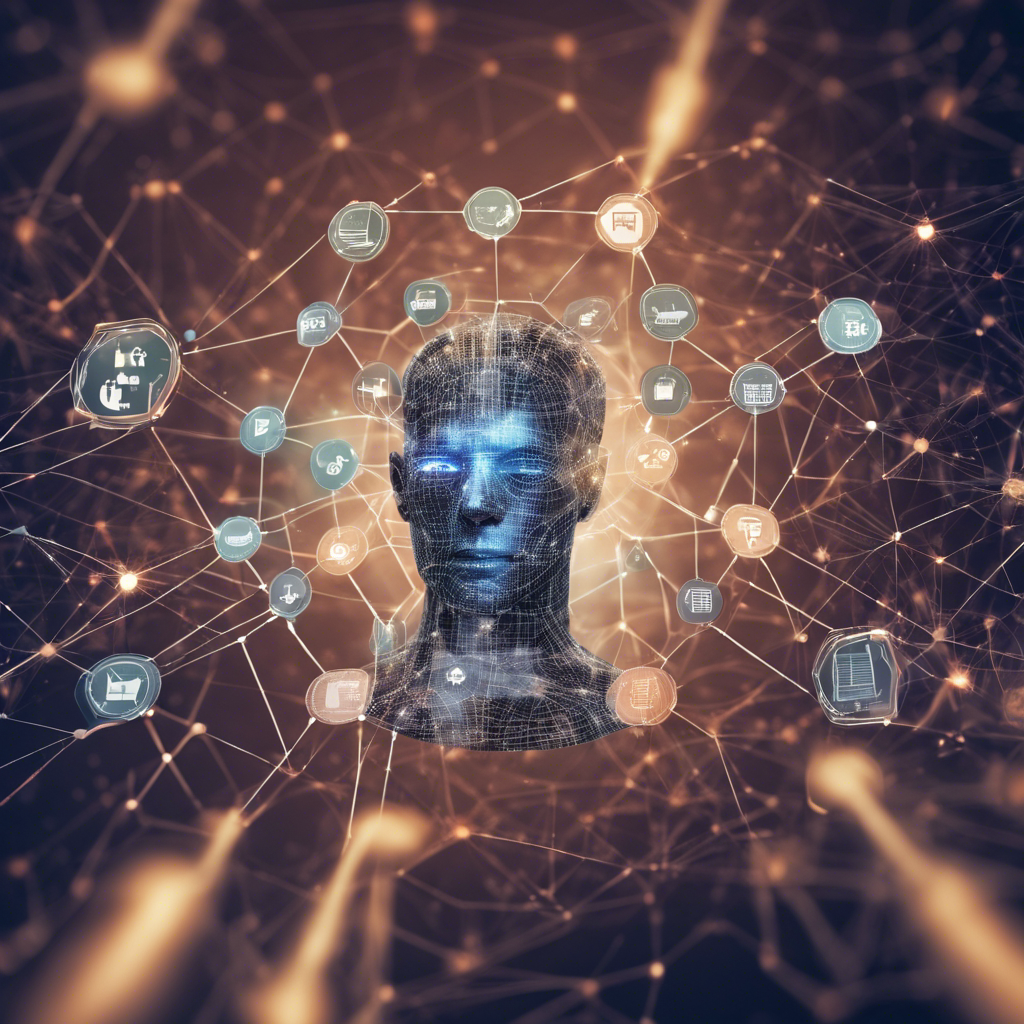
Exploring the Early Impact and Barriers of Generative AI Deployment in the Financial Sector
The advent of generative AI has sparked excitement and speculation across industries, with the potential to revolutionize various sectors. In financial services, where digital tools are already extensively utilized, the impact of generative AI is expected to be significant. McKinsey estimates that generative AI could add trillions of dollars annually to the global economy, with the banking industry poised to see substantial benefits. This report delves into the early applications of generative AI in the financial sector, the challenges faced, and the potential for successful deployment.
Nascent Deployment of Generative AI in Financial Services
While the financial industry has embraced digital tools, the adoption of generative AI is still in its infancy. Currently, the most prevalent use cases involve automating repetitive tasks to reduce costs and free up employees for higher-value work. Generative AI tools are being deployed to streamline processes that previously required humans to analyze unstructured information, saving time and resources.
Limited Commercial Deployment and Ongoing Experimentation
While experimentation with more disruptive generative AI tools is underway, commercial deployment remains rare. Academics and financial institutions are exploring how generative AI can enhance asset selection, simulations, and the understanding of asset correlation and tail risk. However, practical and regulatory challenges hinder widespread implementation. Overcoming these barriers will be crucial for unlocking the full potential of generative AI in financial services.
Legacy Technology and Talent Shortages as Temporary Roadblocks
Legacy technology and data structures pose temporary obstacles to the adoption of generative AI tools, particularly for large banks and insurers. Outdated systems may not be compatible with modern applications. However, digitalization efforts in recent years have alleviated some of these challenges and are expected to continue doing so. Additionally, the scarcity of talent with expertise in generative AI is a hindrance, but financial services companies are investing in training existing staff rather than solely relying on external recruitment. The talent shortage is gradually diminishing, similar to previous technological advancements.
Technological Weaknesses and Regulatory Hurdles
The technology itself presents challenges, as off-the-shelf generative AI tools may not adequately perform complex tasks such as portfolio analysis and selection. Companies must invest time and resources in training their own models to ensure accurate outputs. Validating the complex output from generative AI remains a significant hurdle, with concerns about bias and accountability. Regulatory authorities acknowledge the need for further study on the implications of generative AI and have historically been cautious in approving new tools for widespread use.
Conclusion:
Generative AI holds immense potential for the financial services sector, with the promise of streamlining processes, reducing costs, and unlocking new insights. While deployment is still in its early stages, financial institutions are actively exploring the possibilities and overcoming barriers. Legacy technology and talent shortages are temporary roadblocks that are gradually being addressed. However, challenges related to the technology itself and regulatory approval for specific tasks remain significant hurdles. As the industry continues to navigate these obstacles, the transformative power of generative AI in financial services may soon become a reality.
Download the full report for a deeper understanding of the current landscape and future implications of generative AI in financial services.
Note: This content was produced by Insights, the custom content arm of MIT Technology Review, and not written by MIT Technology Review’s editorial staff.